Multi-Modal AI Outperforms Image-Only Approaches for Mutation Prediction in Colorectal Cancer
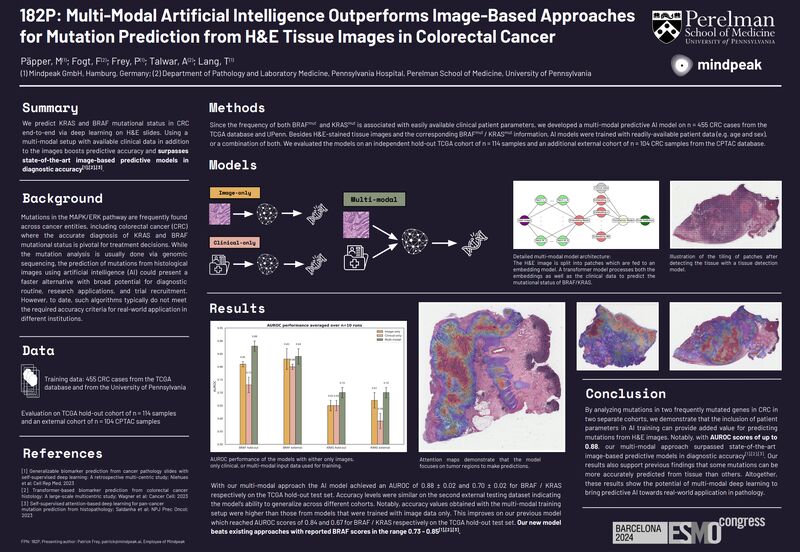
In our latest study in collaboration with University of Pennsylvania Perelman School of Medicine we demonstrate how our multi-modal predictive AI model outperforms image-only approaches for mutation prediction in colorectal cancer.
By combining H&E stained tissue images with clinical patient data for model training, we achieved an impressive AUROC of 0.88 for prediction of BRAF mutations, surpassing state-of-the-art image-based predictive approaches and showcasing the high accuracy and generalizability of our approach. Our findings underscore the transformative impact AI can have in improving diagnostic processes and patient outcomes in oncology.
You may also be interested in
Find out more about our AI-based diagnostics
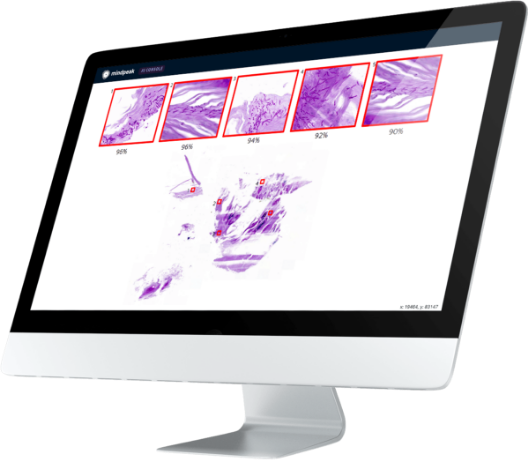